引用本文: | 霍文博,谭英,申万祥,蒋宇扬,陈宇综,陈妍.基于先验知识的分子表征多任务深度卷积神经网络的小样本激酶抑制剂活性预测[J].中国现代应用药学,2022,39(21):2819-2827. |
| HUO Wenbo,TAN Ying,SHEN Wanxiang,JIANG Yuyang,CHEN Yuzong,CHEN Yan.Low Sample Kinase Inhibitory Activity Prediction Capability of Multi-task Deep Convolutional Neural Networks Under Knowledge-based Molecular Representations[J].Chin J Mod Appl Pharm(中国现代应用药学),2022,39(21):2819-2827. |
|
本文已被:浏览 998次 下载 836次 |
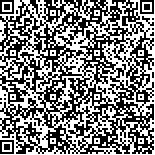 码上扫一扫! |
|
基于先验知识的分子表征多任务深度卷积神经网络的小样本激酶抑制剂活性预测 |
霍文博1, 谭英1, 申万祥2, 蒋宇扬1, 陈宇综3, 陈妍1
|
1.清华大学深圳国际研究生院, 广东 深圳 518055;2.新加坡国立大学, 新加坡 117543;3.深圳湾实验室, 广东 深圳 518000
|
|
摘要: |
为了推动药物研发,深度学习模型被开发用于预测包括激酶在内的各种靶点的抑制剂,且能够达到较好的预测性能。尽管如此,深度学习在小样本靶点(<100个已知的活性化合物)上的预测性能还没有得到充分的测试。本研究利用最近出现的深度卷积神经网络MolMapNet方法在基于先验知识的分子表示下具有的良好的活性预测能力,开发出了多任务MolMapNet模型,用于预测6个激酶亚家族的19个小样本激酶和43个大样本激酶的抑制剂活性。开发的用于所有小样本和大样本激酶的多任务MolMapNet模型明显比单任务模型具有更好的活性预测性能。R2值等活性预测指标在0.651 3~ 0.749 8内表现较好。这证明了多任务迁移学习在小样本靶标活性预测中的鲁棒性。 |
关键词: 深度学习 激酶抑制剂 活性预测 多任务学习 回归策略 药物研发 |
DOI:10.13748/j.cnki.issn1007-7693.2022.21.018 |
分类号:R914.2 |
基金项目: |
|
Low Sample Kinase Inhibitory Activity Prediction Capability of Multi-task Deep Convolutional Neural Networks Under Knowledge-based Molecular Representations |
HUO Wenbo1, TAN Ying1, SHEN Wanxiang2, JIANG Yuyang1, CHEN Yuzong3, CHEN Yan1
|
1.Tsinghua Shenzhen International Graduate School, Shenzhen 518055, China;2.National University of Singapore, Singapore 117543, Singapore;3.Shenzhen Bay Laboratory, Shenzhen 518000, China
|
Abstract: |
To facilitate drug discovery, deep learning models have been developed for the prediction of inhibitors of various targets including kinases, achieving high prediction performances. Nonetheless the ability of deep learning on low-sample targets (<100 known active molecules) has not been adequately tested. Leveraging the good activity prediction capability of a recently emerged deep convolutional neural network MolMapNet method under knowledge-based molecular representations, this study developed multi-task MolMapNet models for inhibitory activity prediction of 19 low-sample kinases and 43 higher-sample kinases of 6 kinase subfamilies. The developed multi-task MolMapNet models for all low-sample and higher-sample kinases significantly enhanced the activity prediction performance over the single-task models. The activity prediction indicators such as R2 values were in the good performance ranges of 0.651 3-0.749 8 for most kinases. These suggest the usefulness of the multi-task transfer learning strategy in activity prediction of low-sample targets. |
Key words: deep learning kinase inhibitors activity prediction multi-task learning regression method drug discovery |