引用本文: | 吴晨璐,王秋悦,所同川,赵静,李正.紫外可见光谱结合化学计量法快速检测双黄连口服液大类成分[J].中国现代应用药学,2023,40(6):736-742. |
| WU Chenlu,WANG Qiuyue,SUO Tongchuan,ZHAO Jing,LI Zheng.Rapid Detection of Major Components in Shuanghuanglian Oral Liquid by UV-Vis Spectroscopy Combined with Chemometrics[J].Chin J Mod Appl Pharm(中国现代应用药学),2023,40(6):736-742. |
|
本文已被:浏览 947次 下载 553次 |
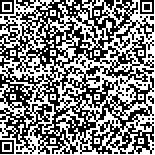 码上扫一扫! |
|
紫外可见光谱结合化学计量法快速检测双黄连口服液大类成分 |
吴晨璐1, 王秋悦1, 所同川1,2, 赵静1,2, 李正1,2
|
1.天津中医药大学中药制药工程学院, 天津 301617;2.省部共建组分中药国家重点实验室, 天津 301617
|
|
摘要: |
目的 基于紫外可见光谱和化学计量学方法建立1种快速检测双黄连口服液质量的方法。方法 采集双黄连口服液紫外可见光谱数据,并使用主成分分析筛选剔除异常样本数据。采用Kennard-Stone算法将所有样本按照7∶3的比例划分为训练集和测试集。采用一阶求导、标准正态变量变换对数据进行预处理,然后结合竞争性自适应重加权采样法提取特征波长,最后将支持向量回归(support vector regression,SVR)、最小二乘支持向量回归(least squares of support vector regression,LS-SVR)、前馈型反向传播(back propagation,BP)神经网络3种方法用于可溶性固形物(soluble solids content,SSC)、总黄酮(total flavones,TF)的定量分析模型的建立。结果 3种模型的决定系数R2均≥0.816 8,均方根误差RMSE均≤4.378 2,均获得了较好的预测效果。对测试集SSC及TF预测结果进行对比发现,与BP神经网络、LS-SVR相比,SVR模型获得了最大R2以及最小RMSE。SVR-SSC模型的R2为0.999 8,RMSE为0.260 3,SVR-TF模型的R2为0.998 3,RMSE为0.543 3。结论 紫外可见光谱结合SVR可以提供1种双黄连口服液质量的高精度快速现场检测方法。 |
关键词: 紫外可见光谱 化学计量法 支持向量回归 最小二乘支持向量回归 BP神经网络 双黄连口服液 质量控制 |
DOI:10.13748/j.cnki.issn1007-7693.20221512 |
分类号:R284.1 |
基金项目:国家自然科学基金项目(81973699,81774148);“重大新药创制”国家科技重大专项(2018ZX09201-011) |
|
Rapid Detection of Major Components in Shuanghuanglian Oral Liquid by UV-Vis Spectroscopy Combined with Chemometrics |
WU Chenlu1, WANG Qiuyue1, SUO Tongchuan1,2, ZHAO Jing1,2, LI Zheng1,2
|
1.College of Pharmaceutical Engineering of Traditional Chinese Medicine, Tianjin University of Traditional Chinese Medicine, Tianjin 301617, China;2.State Key Laboratory of Component Traditional Chinese Medicine, Tianjin 301617, China
|
Abstract: |
OBJECTIVE To study on a rapid method for determining the quality of Shuanghuanglian oral liquid based on UV-Vis spectroscopy and chemometrics. METHODS The UV-Vis spectrum data of Shuanghuanglian oral liquid were collected, and the principal component analysis was used to screen out the abnormal sample data. All samples were segmented into training sets and test sets in a 7:3 ratio using the Kennard-Stone algorithm. The screened data was pre-processed by first derivation and conventional normal variate transform. Then, data feature extraction was performed by competitive adaptive reweighted sampling. Consequently, support vector regression(SVR), least squares to support vector regression(LS-SVR) and back propagation(BP) neural network was used to establish a quantitative analysis model of soluble solids content(SSC) and total flavones(TF). RESULTS All the models achieved good prediction effect, R2 was ≥ 0.816 8, RMSE was ≤ 4.378 2. After comparing the SSC model and the TF model, it was revealed that compared with BP neural network and LS-SVR, the SVR model obtained the highest R2 and the lowest RMSE. The R2 of the SVR-SSC model was 0.999 8, RMSE was 0.260 3, and R2 of SVR-TF model was 0.998 3, RMSE was 0.543 3. CONCLUSION UV-Vis spectrum combined with SVR can provide a high-precision and rapid on-site detection method for the quality of Shuanghuanglian oral liquid. |
Key words: UV-Vis spectrum chemometrics support vector regression least square support vector regression BP neural network Shuanghuanglian oral liquid quality control |