引用本文: | 李君翔,倪琳*,王亚飞,马寒露,宋平顺,杨平荣,王红球.基于多种算法建立牡蛎及石决明的拉曼光谱鉴别模型[J].中国现代应用药学,2023,40(4):477-482. |
| LI Junxiang,NI Lin*,WANG Yafei,MA Hanlu,SONG Pingshun,YANG Pingrong,WANG Hongqiu.Identification Models of Raman Spectrum for Ostreae Concha and Haliotidis Concha Based on Multiple Algorithms[J].Chin J Mod Appl Pharm(中国现代应用药学),2023,40(4):477-482. |
|
本文已被:浏览 1155次 下载 672次 |
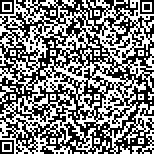 码上扫一扫! |
|
基于多种算法建立牡蛎及石决明的拉曼光谱鉴别模型 |
李君翔1, 倪琳*2, 王亚飞1, 马寒露3, 宋平顺2, 杨平荣2, 王红球3
|
1.兰州大学药学院, 兰州 730000;2.甘肃省药品检验研究院/国家药监局中药材及饮片质量控制重点实验室/甘肃省中藏药检验检测工程技术实验室, 兰州 730000;3.北京鉴知技术有限公司, 北京 100081
|
|
摘要: |
目的 利用拉曼光谱建立多种算法的定性模型,用于贝壳类动物药牡蛎和石决明的鉴别分析。方法 采集32批牡蛎和29批石决明共计366个样品的拉曼光谱,光谱在Matlab软件中作Savitzky-Golay平滑滤波、Scale Normalization for Image Pyramids基线校正、归一化预处理,并对全部及特征波段进行主成分分析降维。使用K最邻近法、决策树算法、判别分析法、集成学习法、支持向量机、人工神经网络6种分类算法建立鉴别模型,通过贝叶斯优化对前5种模型性能进行提升。结果 预处理后拉曼信号峰明显,降维后牡蛎和石决明有聚类趋势。建立以200~310,670~740,1 050~1 100 cm-1为特征波段的5种算法鉴别模型,相比于全波段模型,除集成学习法、支持向量机外,其他算法准确率均有一定的提高。贝叶斯优化后,有3种模型训练集准确率达到98%,测试集准确率达到100%。采用人工神经网络建模分类,训练集、验证集、测试集的正确率均达到100%,能够准确地区分牡蛎和石决明。结论 基于多种算法或IP建立了牡蛎及石决明拉曼光谱鉴别模型,预测效果均较为理想,其中人工神经网络模型可实现牡蛎和石决明的百分百准确、快速鉴别。 |
关键词: 拉曼光谱 分类算法 鉴别模型 牡蛎 石决明 |
DOI:10.13748/j.cnki.issn1007-7693.2023.04.007 |
分类号:R917 |
基金项目:中药材及饮片质量控制重点实验室项目(2021GSMPA-KL01,2021GSMPA-KL02) |
|
Identification Models of Raman Spectrum for Ostreae Concha and Haliotidis Concha Based on Multiple Algorithms |
LI Junxiang1, NI Lin*2, WANG Yafei1, MA Hanlu3, SONG Pingshun2, YANG Pingrong2, WANG Hongqiu3
|
1.School of Pharmacy, Lanzhou University, Lanzhou 730000, China;2.Gansu Provincial Institute of Drug Control/Key Laboratory of Quality Control of Chinese Medicinal Materials and Decoction Pieces of NMPA/Gansu Province Chinese and Tibetan Medicine Testing Engineering Technology Laboratory, Lanzhou 730000, China;3.JINSP Co., Ltd., Beijing 100081, China
|
Abstract: |
OBJECTIVE To establish qualitative models of Raman spectroscopy for identification of shellfish animal drugs Ostreae Concha and Haliotidis Concha based on multiple algorithms. METHODS The Raman spectrograms of 366 samples from 32 batches of Ostreae Concha and 29 batches of Haliotidis Concha were collected respectively. The spectrograms were processed by Savitzky-Golay smoothing filter, Scale Normalization for Image Pyramids, and normalized preprocessing in Matlab. Principal component analysis was used to reduce dimension of full band and specific bands. Six classification algorithms including K-nearest neighbor, decision tree, discriminant analysis, ensemble learning, support vector machine and artificial neural network were used to establish the identification models, and the performance of the first five models was improved by Bayesian optimization. RESULTS The Raman signal peaks were obvious after preprocessing, and there was obvious clustering trend of Ostreae Concha and Haliotidis Concha after dimension reduction. Five algorithmic identification models with 200-310, 670-740 and 1050-1100 cm-1 as characteristic bands were established. Compared with the full band model, the accuracy of all classifiers were improved except ensemble learning and support vector machines. After Bayesian optimization, the accuracy of three models achieved 98% in training set and 100% in test set. Using artificial neural network modeling for classification, the accuracy of training set, validation set and test set reached 100%, which could distinguish Ostreae Concha and Haliotidis Concha well. CONCLUSION Qualitative models of multiple algorithms by Raman spectroscopy for the identification of Ostreae Concha and Haliotidis Concha are established. The predictions of each model are satisfactory. Among them, the artificial neural network model can achieve 100% accurate and fast identification of Ostreae Concha and Haliotidis Concha. |
Key words: Raman spectroscopy classification algorithm identification model Ostreae Concha Haliotidis Concha |